Publications #
Ridge prediction under dense factor augmented models #
Yi He
Accepted by Journal of the American Statistical Association. AI Percentile:98%

Paper (Open Access) Supplementary MaterialsThis paper establishes a comprehensive theory of the optimality, robustness, and cross-validation selection consistency for the ridge regression under factor-augmented models with possibly dense idiosyncratic information. Using spectral analysis for random matrices, we show that the ridge regression is asymptotically efficient in capturing both factor and idiosyncratic information by minimizing the limiting predictive loss among the entire class of spectral regularized estimators under large-dimensional factor models and mixed-effects hypothesis. We derive an asymptotically optimal ridge penalty in closed form and prove that a bias-corrected k-fold cross-validation procedure can adaptively select the best ridge penalty in large samples. We extend the theory to the autoregressive models with many exogenous variables and establish a consistent cross-validation procedure using the what-we-called double ridge regression method. Our results allow for non-parametric distributions for, possibly heavy-tailed, martingale difference errors and idiosyncratic random coefficients and adapt to the cross-sectional and temporal dependence structures of the large-dimensional predictors. We demonstrate the performance of our ridge estimators in simulated examples as well as an economic dataset. All the proofs are available in the supplement, which also includes more technical discussions and remarks, extra simulation results, and useful lemmas that may be of independent interest.
Extreme value inference for heterogeneous power law data #
John H.J. Einamhl, Yi He
Accepted by The Annals of Statistics. AI Percentile: 98%
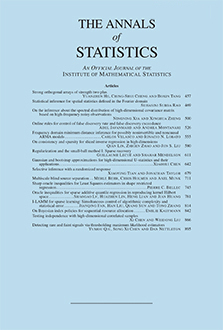
Paper (Open Access)We extend extreme value statistics to independent data with possibly very different distributions. In particular, we present novel asymptotic normality results for the Hill estimator, which now estimates the extreme value index of the average distribution. Due to the heterogeneity, the asymptotic variance can be substantially smaller than that in the i.i.d. case. As a special case, we consider a heterogeneous scales model where the asymptotic variance can be calculated explicitly. The primary tool for the proofs is the functional central limit theorem for a weighted tail empirical process. A simulation study shows the good finite-sample behavior of our limit theorems. We also present applications to assess the tail heaviness of earthquake energies and of cross-sectional stock market losses.
Extreme value estimation for heterogeneous data #
John H.J. Einmahl and Yi He
Journal of Business & Economic Statistics, 41:1, 255-269, 2023. AI Percentile: 98%

Paper (Open Access) MATLAB codesWe develop a universal econometric formulation of empirical power laws possibly driven by parameter heterogeneity. Our approach extends classical extreme value theory to specifying the tail behavior of the empirical distribution of a general data set with possibly heterogeneous marginal distributions. We discuss several model examples that satisfy our conditions and demonstrate in simulations how heterogeneity may generate empirical power laws. We observe a cross-sectional power law for US stock losses and show that this tail behavior is largely driven by the heterogeneous volatilities of the individual assets.
Most powerful quadratic tests for a sequence of high dimensional local alternatives #
Yi He, Sombut Jaidee and Jiti Gao
Journal of Econometrics, 234:1, 151-177, 2023. AI Percentile: 96%

Paper (Open Access) MATLAB codesWe develop a powerful quadratic test for the overall significance of many covariates in a dense regression model in the presence of nuisance parameters. By equally weighting the sample moments, the test is asymptotically correct in high dimensions even when the number of coefficients is larger than the sample size. Our theory allows a non-parametric error distribution and weakly exogenous nuisance variables, in particular autoregressors in many applications. Using random matrix theory, we show that the test has the optimal asymptotic testing power among a large class of competitors against local alternatives whose coordinates are dense in the eigenbasis of the high dimensional sample covariance matrix among regressors. The asymptotic results are adaptive to the covariates’ cross-sectional and temporal dependence structure and do not require a limiting spectral law of their sample covariance matrix. In the most general case, the nuisance estimation may play a role in the asymptotic limit and we give a robust modification for these irregular scenarios. Monte Carlo studies suggest a good power performance of our proposed test against high dimensional dense alternative for various data generating processes. We apply the test to detect the significance of over one hundred exogenous variables in the FRED-MD database for predicting the monthly growth in the US industrial production index.
Risk Analysis via Generalized Pareto Distributions #
Yi He, Liang Peng, Dabao Zhang and Zifeng Zhao
Journal of Business & Economic Statistics, 40:2, 852-867, 2022. AI Percentile: 98%
This paper is listed among the most read article (all time) at JBES

Paper (Open Access) R CodesWe compute the value-at-risk of financial losses in the tail by fitting a generalized Pareto distribution to exceedances over a high but not divergent threshold. This paper infers such a model for both independent observations and time series data. We show that the asymptotic variance for the maximum likelihood estimation depends on the choice of threshold unlike the existing study of using a divergent threshold. For interval estimation, we propose a random weighted bootstrap method with critical values computed by the empirical distribution of the absolute differences between the bootstrapped estimators and the maximum likelihood estimator. The finite sample performance of the derived confidence intervals is demonstrated through numerical studies before applying to real data in insurance and finance.
Inference for conditional value-at-risk of a predictive regression #
Yi He, Yanxi Hou, Liang Peng and Haipeng Shen
The Annals of Statistics, 48:6, 3442-3464, 2020. AI Percentile: 98%
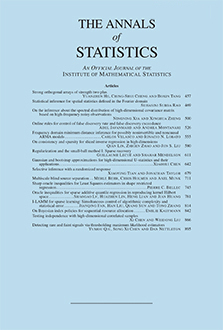
Paper Accepted VersionConditional value-at-risk is a popular risk measure in risk management. We study the inference problem of conditional value-at-risk under a linear predictive regression model. We derive the asymptotic distribution of the least squares estimator for the conditional value-at-risk. Our results relax the model assumptions made in Chun et al. (2012) and correct their mistake in the asymptotic variance expression. We show that the asymptotic variance depends on the quantile density function of the unobserved error and whether the model has a predictor with infinite variance, which makes it challenging to actually quantify the uncertainty of the conditional risk measure. To make the inference feasible, we then propose a smooth empirical likelihood based method for constructing a confidence interval for the conditional value-at-risk based on either independent errors or GARCH errors. Our approach not only bypasses the challenge of directly estimating the asymptotic variance but also does not need to know whether there exists an infinite variance predictor in the predictive model. Furthermore, we apply the same idea to the quantile regression method, which allows infinite variance predictors and generalizes the parameter estimation in Whang (2006) to conditional value-at-risk in the supplementary material. We demonstrate the finite sample performance of the derived confidence intervals through numerical studies before applying them to real data.
Statistical inference for a relative risk measure #
Yi He, Yanxi Hou, Liang Peng and Jiliang Sheng
Journal of Business & Economic Statistics, 37:2, 301-311, 2019. AI Percentile: 98%

Paper R codesFor monitoring systemic risk from regulators’ point of view, this article proposes a relative risk measure, which is sensitive to the market comovement. The asymptotic normality of a nonparametric estimator and its smoothed version is established when the observations are independent. To effectively construct an interval without complicated asymptotic variance estimation, a jackknife empirical likelihood inference procedure based on the smoothed nonparametric estimation is provided with a Wilks type of result in case of independent observations. When data follow from AR-GARCH models, the relative risk measure with respect to the errors becomes useful and so we propose a corresponding nonparametric estimator. A simulation study and real-life data analysis show that the proposed relative risk measure is useful in monitoring systemic risk.
Estimation of extreme depth-based quantile regions #
Yi He and John H.J. Einmahl
Journal of the Royal Statistical Society - Series B , 79:449-461, 2017. AI Percentile: 99%
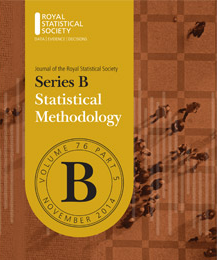
Consider the extreme quantile region induced by the half‐space depth function HD of the form \( \mathcal{Q}=\{x\in\mathbb{R}^d:HD(x,P)\leq \beta\}, \) such that \( P\mathcal{Q}=p \) for a given, very small p>0. Since this involves extrapolation outside the data cloud, this region can hardly be estimated through a fully non‐parametric procedure. Using extreme value theory we construct a natural semiparametric estimator of this quantile region and prove a refined consistency result. A simulation study clearly demonstrates the good performance of our estimator. We use the procedure for risk management by applying it to stock market returns.